…and natural language processing
linguistics & NLP | physics | geography
Georgetown University Linguistics
I am currently a PhD student at Georgetown Linguistics (2024 – ), surveying a general topic: human and computational representations of structure in language.
- Common ground inventory modeling with syntactic, semantic, and information structure annotations as additions to the Georgetown University Multilayer corpus (Zeldes et al. 2017). Advised by Amir Zeldes.
- Universal Dependencies compatibility updates to the Korean SNACS (Sentiment Network of Adposition and Case Supersenses) dataset (Hwang et al. 2020). Advised by Nathan Schneider.
- Statistical investigations into prosody-syntax interface: prosody as cues of syntactic structure and syntax as elements of prosodic design, following Wolf et al. (2023). Advised by Ethan G. Wilcox.
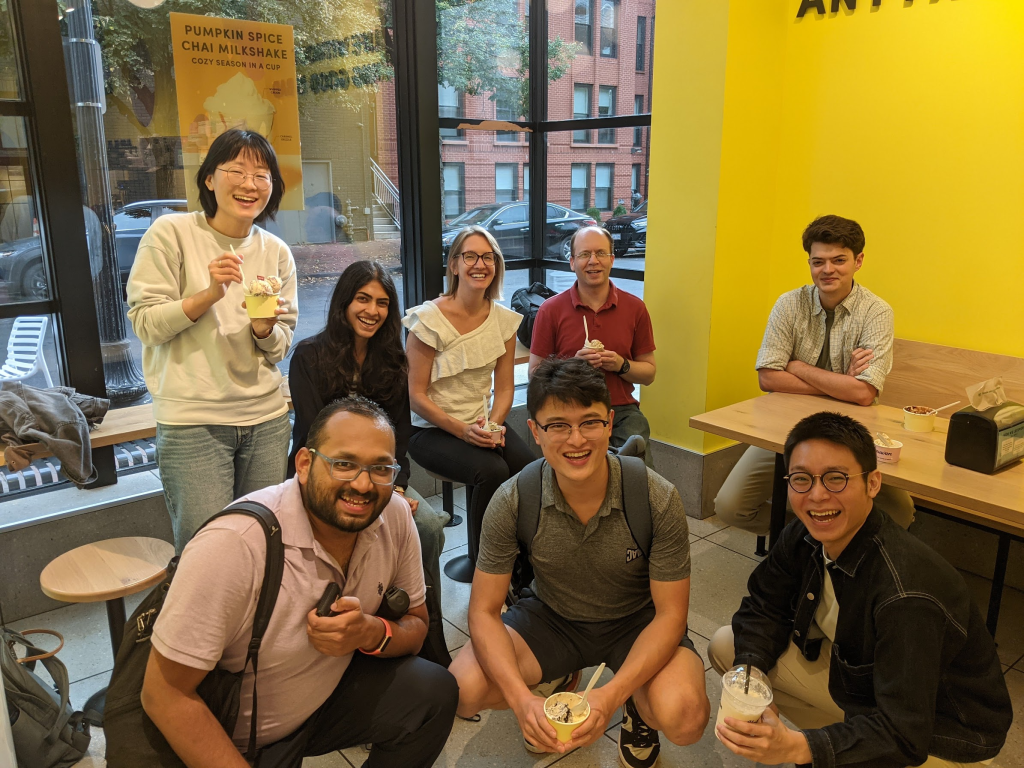
NCSOFT
At NCSOFT (2021 – 2024), I primarily worked on structure-related tasks like chunking, information extraction, salience measurement, and literary understanding. My projects included:
Natural language understanding, Language AI Lab
- Concatenation of light embeddings yields a fast, light, and effective chunking system capable of processing up to 10k requests per seconds on less than 4GB of GPU memory (2021).
- Set of 10 naïve rules applied on training set significantly improves resulting information extraction model performance (2021 – 22).
Financial language understanding, NLP Center
- An ensemble of rule- and transformer-based noise detection system to improve open information extraction (Goldie and Min, 2022).
- A simple TF-IDF based importance metric effectively ranks events within a temporal window (2022 – 23).
- Large language models can be used to augment relation classification datasets and improve out-of-distribution, out-of-domain performance (Kim and Min, 2023).
- Punctuation restoration as unsupervised representation learning objective improves structure understanding (2023). tech blog (Korean) poster
- Modifications in loss and decoding can yield a structure prediction model from a natural language generation model (Lee, Min, Lee, and Lee 2023). arXiv
Creative AI, Research & Innovation
- Computational processing of literary devices and related baseline performances (2024).
wecommit
If ChatGPT is so good, why don’t we delegate our paperwork? Accelerated by Antler, wecommit is our attempt at narrow-domain paperwork automation.
forus.ai
If you have some hot takes on whether AI and art mix well, I’ve developed an AI songwriter with forus.ai. We got an artist to rap an excerpt–you can listen to it here.
Johns Hopkins University Cognitive Science
At JHU Cognitive Science (2019 – 2020), I worked with with Tom McCoy and Tal Linzen to evaluate and improve syntactic generalization abilities in language models.
- BERT fine-tuned on MNLI and is unstable and vulnerable to syntactic heuristics (McCoy, Min, Linzen 2021).
- Adversarial data augmentation via syntactic manipulation of training set data significantly increases robustness to augmentation-like examples and general syntactic sensitivity too (Min, McCoy, Das, Pitler, Linzen 2020).
- Heuristics likely arise from both the pre-training and the fine-tuning dataset. Currently popular fine-tuning and evaluation paradigm has drawbacks that can be patched with longer fine-tuning on unbiased datasets, multi-seed out-of-distribution evaluation, and syntactic adversarial augmentation (Master’s thesis).
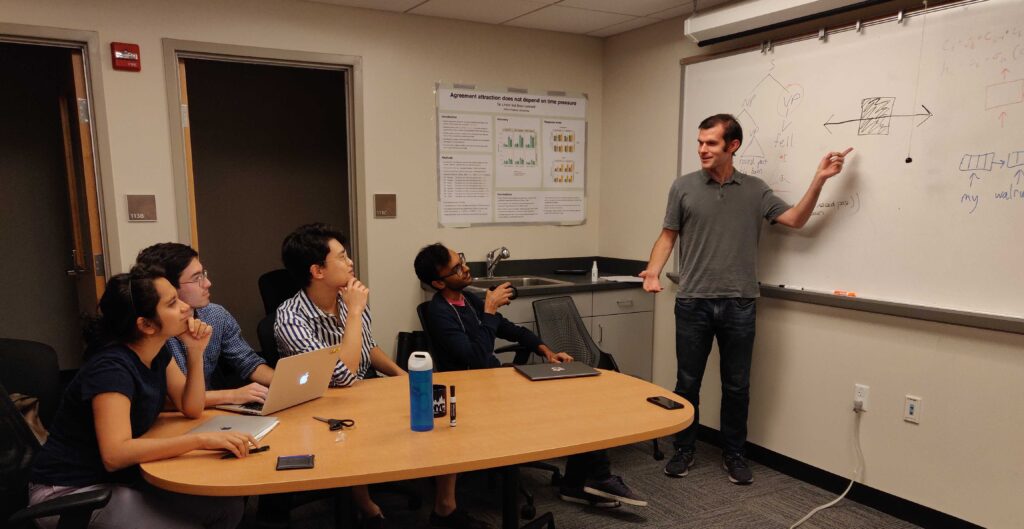